The Internet of Things (IoT) is destined to transform not only our daily lives but the entire fabric of human society. From smart homes to the factories of the future, the number of connected devices continues to increase at a rapid rate. According to IDC, there will be more than 55.7 B connected devices by 2025, 75% of which will be linked to an IoT platform. This will lead to an outflux of data generated by these devices, growing from 18.3 ZB in 2019 to an estimated 73.1 ZB by 2025. At this point, the integration of efficient Artificial Intelligence (AI) algorithms will present numerous ways to overcome some of the challenges of IoT deployments. Advancements in both AI and IoT devices have ensured a systematic computational analysis of data which in turn has resulted in overcoming challenges such as latency and security breaches. It has also succeeded in enabling real-time data processing ability, whilst creating dynamic networks and distributed intelligence.
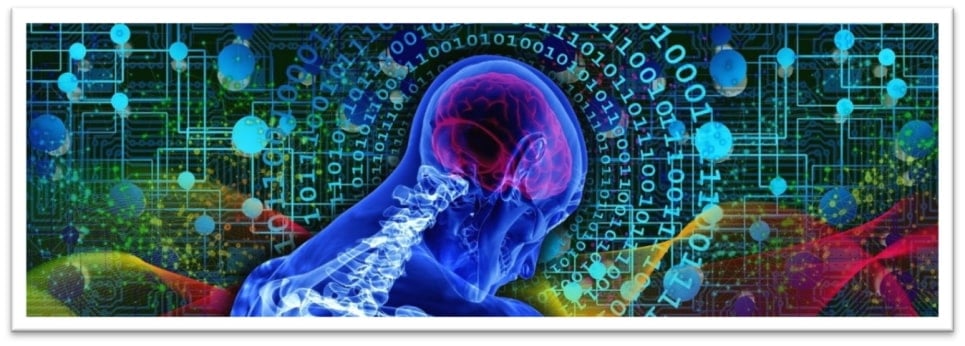
What is AIoT?
Artificial Intelligence of Things (AIoT) is a relatively new term that is currently predominating the world. The terminology is the combination of the two big giants in emerging technologies-Artificial Intelligence (AI) and the Internet of Things (IoT), to achieve more efficient IoT operations, improve human-machine interactions and enhance data management and analytics. IoT is comprised of interconnected things that have sensors within which can collect or generate a huge amount of data from the environment. AI provides the possibility of machines learning from previous experiences to perform tasks based on their prior knowledge. AI can convert IoT data into useful information, which can then be used efficiently in making suitable decisions at the appropriate time. AI and IoT both share a reciprocal relationship - AI prospers from the availability of “big data”, connectivity, and signals from IoT, whereas IoT benefits from machine learning capabilities.
In AIoT, AI is embedded in the IoT infrastructure components, and API is used to achieve interoperability and reliability between these components in many layers. This operational form focuses on improving the system and network functionality and operations, as well as managing data to extract the hidden value. Data analytics has increased in value with additional intelligence due to the combination of AI, IoT, and the systems. For instance, the data from the edge of the IoT network automates machines and enables them to activate tasks at the operational level. To provide a clearer explanation of where AI and IoT converge, a data life cycle is shown in the next figure. The IoT takes the responsibility of capturing the data using sensors in the IoT perception layer. It then communicates it within the network layer, followed by aggregating the data to ensure integration. The data is then analyzed through the IoT platform. Finally, based on the results of the analysis, the “Act” is performed on the application/business layer. The essential value of the IoT is determined by the value of “Act” in the application layer, which depends mainly on the previous step “Analysis”. This step “Analysis” is the playground of AI.
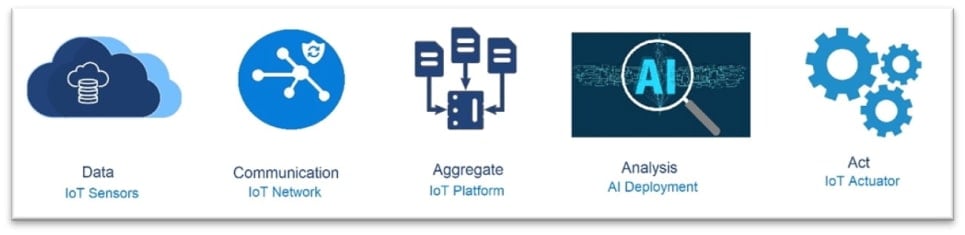
AI: A Real Value-add to IoT
Integration of AI into the IoT network from the cloud specifically at the endpoint provides the ability to improve the operational status and overall system efficiency, supporting IoT scalability and mitigating risk by effective risk management. This would help reduce operational costs by minimizing urgent downtime, which in turn means improved availability.
The efficient AI inference at the embedded endpoint demands a revolutionary way of managing information, accumulating relevant data, and making decisions locally on the device.
In order to adopt effective Artificial intelligence (AI), Machine Learning (ML), or Deep Learning (DL) at the endpoint, you need convenient hardware that can handle the core system tasks as well as related algorithms which will not compromise on performance or power consumption. At this point, the IoT-ready microcontrollers available with required CPU performance, intelligent power-saving peripherals and more importantly robust hardware security engines can help scale the AIoT adoption. Nevertheless, hardware solely on its own is not the answer as we need optimized middleware supporting intelligent ML models to be able to run on these constrained devices.
Smart IoT devices based on IoT-ready microcontroller units working at the edge of the IoT network are responsible for collecting and processing huge amounts of data. The operation of processing and transferring data from the edge to the cloud demands optimized performance, secure communication and above all high energy consumption. Keeping the energy consumption at a very low level is an important requirement for the deployment of IoT devices at scale. Here TinyML provides techniques to optimize and run highly complex AI/ML models effectively and ecologically.
Let’s now look deeper into the evolution and enablement of TinyML at the endpoint and AIoT adoption at different stages.
Stage 1: AI and the Cloud:
This is the starting point, where the cloud is the only place for training and hosting machine learning models, ultimately requiring greater power for computing operations as well as application-related task management. The microcontroller units are responsible for managing the sensors and actuators in the area of an IoT network. As shown in the figure below, AI models are processed on the cloud where the IoT network may be overloaded while transmitting the data to the cloud. All of this will introduce latency and consume resources, making it particularly unsuitable for real-time and safety-critical applications.
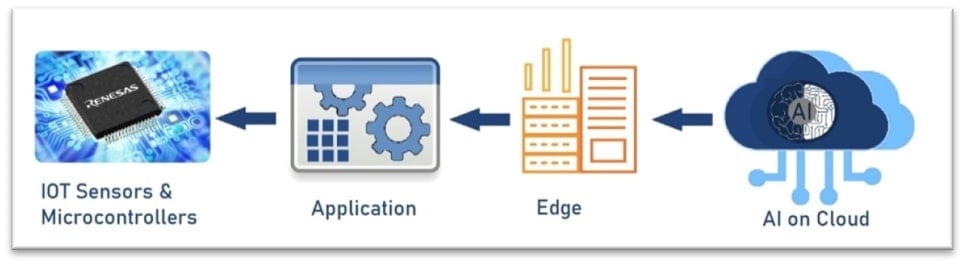
Stage 2: AI and the Edge:
To improve system efficiency and decision making, we now have the ability to run AI models locally on the edge or endpoint. However, with resource-constrained edge devices, the training of the machine learning models still needs to happen in the cloud. The trained models can then be deployed at the edge for execution. This approach benefits from the power of the cloud (training), and the low latency on the edge (faster execution).
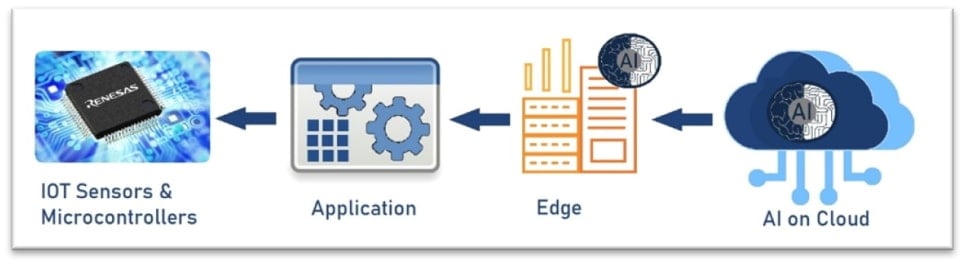
Stage 3: Embedding Intelligence at the Endpoint:
In this stage, designers of the IoT products can embed machine learning models directly onto the microcontrollers; skipping the extra step of any additional ‘act’ on the edge of the cloud.
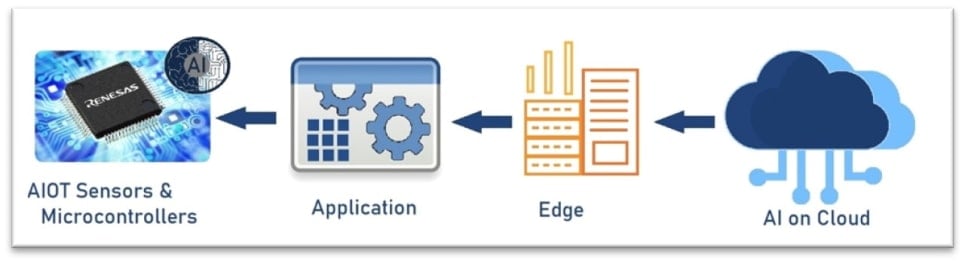
However, such ML models running on MCUs must be lightweight enough to enable faster execution. This is where TinyML comes into play as it doesn’t need many resources to work making it a convenient solution for IoT constraint devices. In short, TinyML helps to optimize resources, reduce cost of ownership, increase energy efficiency, improve data security, and ultimately lower latency. This gives birth to distributed intelligence and will be the catalyst to scale AIoT adoption across a wide variety of application segments.
Let’s look at some use cases where Renesas can help achieve a highly efficient AIoT solution; for example, predictive maintenance in the industrial segment where embedding relevant machine learning models will help to detect any problems in real time and act accordingly.
Pattern Recognition: This is an image or speech-recognition model that can alter the system when detecting certain words or instructions.
Smart Healthcare: Applications include intelligent pre-diagnostics, medical condition monitoring and real-time analysis, effective image recognition for speedy treatment, and more.
Wearable Smart Devices: These applications are endless ranging from smartwatches to fitness trackers and a variety of monitoring devices.
The advent of AIoT is spanning across many new technologies and applications, opening the opportunity for unprecedented progress in all sectors of human life. Wider adoption of AIoT calls for collaboration and standardization in terms of connectivity, security and development of advanced technology to break down any barriers.
The path to the development of billions of smart devices is opening up wide and creating new applications and business streams that were not possible before. Unleash your imagination as what is to come is beyond expectations.