Renesas is a leading supplier of MCUs, shipping more than 3.5 billion devices each year backed by a dual-source production model, the industry’s most advanced MCU process technology and a network of more than 200 ecosystem partners. Approximately half of Renesas MCUs are designed into automotive platforms, with the remainder serving a diverse set of applications in IoT, edge-to-cloud computing, consumer, industrial and infrastructure systems. Veteran semiconductor industry editor and consultant, Andrew MacLellan, recently sat down with Roger Wendelken, Senior Vice President of the MCU Business Division in Renesas’ IoT and Infrastructure Business Unit, to discuss the evolving MCU market, thoughts on nurturing a user-friendly customer design ecosystem and the impact of artificial intelligence (AI) on MCU component selection and design flows.
Andrew: What are the major trends influencing the MCU landscape for industrial applications?
Roger: One is to address the emerging AI markets of real-time analytics, vision and voice. The move to embedded AI, or what we call “endpoint intelligence,” is driving the need for higher-performance MCU cores that bring in DSP functionality as well as hardware acceleration engines and neural nodes.
A second key trend is that today's engineer would love to design everything over the Internet – and do it very easily. That requires a user interface that provides engineers with a seamless design flow. Frankly, this trend is directly in line with the overriding goal of Renesas, “To Make Our Lives Easier” by complementing human capabilities. If they become frustrated with the documentation, or they can’t find an evaluation kit, or the app notes or software manuals are hard to follow, then they always have the option of locking in with a competitor.
Andrew: How are you enabling customers to embrace these trends, and how are you helping them manage the growing size and complexity of the MCU ecosystem?
Roger: It's a combination of ease of design, helping them do more work remotely and creating an environment where, say, they can play with an eval kit in the cloud as opposed to buying a physical kit and setting it up. In the cloud, there’s no set up required; it's there already for designers to take measurements and do benchmarking. That was the impetus behind the recent launch of our Quick-Connect Studio, which is a cloud-based design platform that lets users graphically build hardware and software and accelerate product development by quickly validating prototypes.
Secondly, because the ecosystem is becoming so complex, we break it into several subsegments, such as security and safety, AI, tools and user experience, connectivity and cloud, and human machine interface sensing and control. Then, we pick ecosystem partners to support each subsegment. Of those, AI is probably the most complex given the need to build, develop, train and deploy models. There could be ecosystem partners for each of those different categories. Reality AI, which we acquired last year, was initially an AI ecosystem partner, and we saw a tremendous benefit for them to become part of Renesas and our overall customer solution.
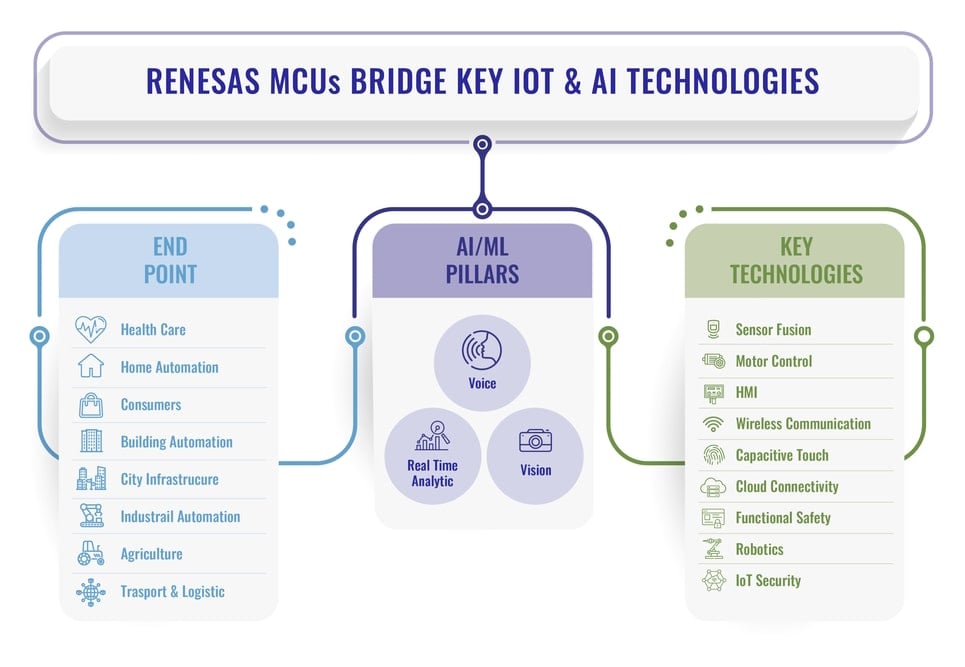
Andrew: I read a report recently that debated whether the move to incorporate multiple, highly optimized cores and AI algorithms will result in general-purpose MCUs being replaced by application-specific devices. What’s your take?
Roger: I wouldn’t necessarily agree that they’re becoming more application specific, and I'll give you my reasoning on this. At Renesas, we have both, and what really differentiates the two is the hardware peripherals. For example, the metering or motor control markets may require certain analog functions that aren’t needed in a general-purpose MCU for someone designing for other applications. That necessitates a trade-off between die size and cost.
Why is that becoming less of an issue? It’s because, as we move to lithographies of 22nm and below, you can start putting a lot more functionality into a single piece of silicon that can now address many segments without incurring a heavy cost penalty. In a sense, as you move to smaller lithographies, you can almost create a general-purpose MCU that is able to address a lot of application-specific designs through different packaging schemes and memory size options. For example, our most advanced 32-bit MCUs have very powerful cores with the ability to handle all aspects of AI, from real-time analytics to vision and voice. But you can also take the IP on the die and bond it out into different packages that can address the networking market, or metering or motor control.
Andrew: With more MCUs being designed into the IoT intelligent endpoint, how is Renesas helping customers manage security?
Roger: As more and more products are connected to the cloud via home and industrial networks, we need to ensure that the network and endpoint are secure. AI/ML (machine learning) is a powerful technology, and it’s important not to underestimate its implied security requirements. Protecting AI IP and device cloud communication – efficiently and cost effectively – is vital, but new technology also introduces new threats. This is why governments around the world are responding with legislation such as the Strengthening American Cybersecurity Act and the EU Cyber Resilience Act. These are designed to defend users and their devices from malicious attack. Protecting the AI model, during both training and operation, is critical to secure operation of the device over its entire lifetime.
Andrew: What effect are AI and ML/TinyML having on the way MCUs are designed in at the system level?
Roger: One thing we've learned is that, when a customer implements AI into their product, there is a direct impact on the MCU selection decision, which now needs to happen far earlier in the design process. This is because customers have to decide how big their model needs to be based on the capabilities they’re designing for. All of that impacts what MCU they need to run the model, the optimal memory density and what performance is required to achieve the goals of their AI application. If I can show my customer how they can implement AI on a 50-cent MCU using my ecosystem and my tools versus a 75-cent MCU from a competitor, that’s what it comes down to. This places a heavy reliance on how tightly these algorithms are written so that they can be executed using fewer resources, and again that’s where Reality AI comes in as a winning solution.
Andrew: What advice do you have for customers at the initial stages of their design process?
Roger: For a product that’s entering design today and will hit the market in a year or two and stay relevant for the next five, engineers need to think about using security and AI to future-proof their product so it remains competitive for its full life cycle.
For more information, please visit: https://www.renesas.com/products/microcontrollers-microprocessors