Sensors are increasingly being used in our everyday lives to help collect meaningful data across a wide range of applications, such as building HVAC systems, industrial automation, healthcare, access control, and security systems, just to name a few. Sensor Fusion network assists in retrieving data from multiple sensors to provide a more holistic view of the environment around a smart endpoint device. In other words, Sensor Fusion provides techniques to combine multiple physical sensor data to generate accurate ground truth, even though each individual sensor might be unreliable on its own. This process helps to reduce the amount of uncertainty that may be involved in overall task performance.
To increase intelligence and reliability, the application of deep learning for sensor fusion is becoming progressively important across a wide range of industrial and consumer segments.
From a data science perspective, this paradigm shift allows extracting relevant knowledge from monitored assets through the adoption of intelligent monitoring and sensor fusion strategies, as well as by the application of machine learning and optimization methods. One of the main goals of data science in this context is to effectively predict abnormal behavior in industrial machinery, tools, and processes to anticipate critical events and damage, eventually preventing important economic losses and safety issues.
Renesas Electronics provides intelligent endpoint sensing devices as well as a wide range of analog rich Microcontrollers that can become the heart of smart sensors, which enable a more accurate sensor fusion solution across different applications. In this context combining sensor data in a typical sensor fusion network may be achieved as follows:
- Redundant sensors: All sensors give the same information to the world.
- Complementary sensors: The sensors provide independent (disjointed) types of information about the world.
- Coordinated sensors: The sensors collect information about the world sequentially.
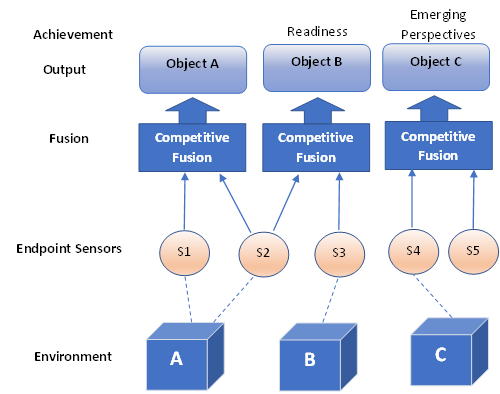
The communication in a sensor network is the backbone of the entire solution and could be in any of the schemes mentioned below:
- Decentralized: No communication exists between the sensor nodes.
- Centralized: All sensors provide measurements to a central node.
- Distributed: The nodes interchange information at a given communication rate (e.g., every five scans, i.e., one-fifth communication rate).
The centralized scheme can be regarded as a special case of the distributed scheme where the sensors communicate every scan to each other. A pictorial representation of the fusion process is given in the figure below.
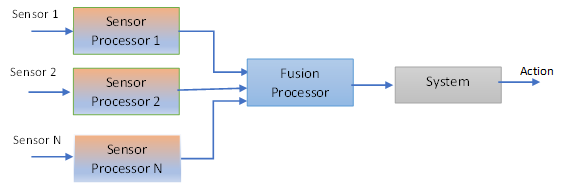
From Industry 4.0 perspective, feedback from one sensor is typically not enough, particularly for the implementation of control algorithms.
Deep Learning
Precisely calibrated and synchronized sensors are a precondition for effective sensor fusion. Renesas provides a range of solutions to enable informed decision-making by executing advanced sensor fusion at the endpoint on a centralized processing platform.
Performing late fusion allows for interoperable solutions, while early fusion gives AI rich data for predictions. Leveraging the complementary strengths of different strategies gives us the key advantage. The modern approach involves time and space synchronization of all onboard sensors before feeding synchronized data to the neural network for predictions. This data is then used for AI training or Software-In-the-Loop (SIL) testing of real-time algorithm that receives just a limited piece of information.
Deep learning involves the use of neural networks for the purpose of advanced machine learning techniques that leverage high-performance computational platforms such as Renesas RA MCU and RZ MPU for enhanced training and execution. These deep neural networks consist of many processing layers arranged to learn data representations with varying levels of abstraction from sensor fusion. The more layers in the deep neural network, the more abstract the learned representations become.
Deep learning offers a form of representation learning that aims to express complicated data representations by using other simpler representations. Deep learning techniques can understand features using a composite of several layers, each with unique mathematical transforms, to generate abstract representations that better distinguish high-level features in the data for enhanced separation and understanding of true form.
Multi-stream neural networks are useful in generating predictions from multi-modal data, where each data stream is important to the overall joint inference generated by the network. Multi-stream approaches have been shown successful for multi-modal data fusion, and deep neural networks have been applied successfully in multiple applications such as neural machine translation and time-series sensor data fusion.
This is a tremendous breakthrough that allows deep neural networks to train and deploy on MCU-based Endpoint applications, thereby helping to accelerate industrial adoption. Renesas RA MCU platform and associated Flexible SW Package combined with AI modeling tools offer the ability to apply many of the neural network layers as a multi-layer structure. Typically, more layers lead to more abstract features learned by the network. It has been proven that stacking multiple types of layers in a heterogeneous mixture can outperform a homogeneous mixture of layers. Renesas sensing solutions can be used to compensate for deficiencies in information by utilizing feedback from multiple sensors. The deficiencies associated with individual sensors to calculate types of information can be compensated for by combining the data from multiple sensors.
The flexible Renesas Advanced (RA) Microcontrollers (MCUs) are industry-leading 32-bit MCUs and are a great choice for building smart sensors. With a wide range of Renesas RA family MCUs, you can choose the best one as per your application needs. The Renesas RA MCU platform, combined with strong support & SW ecosystem, will help accelerate the development of Industry 4.0 applications with sensor fusion and deep learning modules.
As part of Renesas' extensive solution and design support, Renesas provides a reference design for a versatile Artificial Internet of Things (AIoT) sensor board solution. It targets applications in industrial predictive maintenance, smart home/IoT appliances with gesture recognition, wearables (activity tracking), and mobile for innovative human-machine interface, or HMI, (FingerSense) solutions. As part of this solution, Renesas can provide a complete range of devices, including an IoT-specified RA microcontroller, air quality sensor, light sensor, temperature and humidity sensor, a 6-axis inertial measurement unit as well as Cellular and Bluetooth communication support.
With the increasing number of sensors in Industry 4.0 systems comes a growing demand for sensor fusion to make sense of the mountains of data that those sensors produce. Suppliers are responding with integrated sensor fusion devices. For example, an intelligent condition monitoring box is available designed for machine condition monitoring based on fusing data from vibration, sound, temperature, and magnetic field sensors. Additional sensor modalities for monitoring acceleration, rotational speeds, and shock and vibration can be included optionally.
The system implements sensor fusion through AI algorithms to classify abnormal operating conditions with better granularity resulting in high probability decision making. This edge AI architecture can simplify handling the big data produced by sensor fusion, ensuring that only the most relevant data is sent to the edge AI processor or to the cloud for further analysis and possible use in training ML algorithms.
The use of AI-based Deep Learning has several benefits:
- The AI algorithm can employ sensor fusion to utilize the data from one sensor to compensate for weaknesses in the data from other sensors.
- The AI algorithm can classify the relevance of each sensor to specific tasks and minimize or ignore data from sensors determined to be less important.
- Through continuous training at the edge or in the cloud, AI/ML algorithms can learn to identify changes in system behavior that were previously unrecognized.
- The AI algorithm can predict possible sources of failures, enabling preventative maintenance and improving overall productivity.
Sensor fusion combined with AI deep learning produces a powerful tool to maximize the benefits when using a variety of sensor modalities. AI/ML-based enhanced sensor fusion can be employed at several levels in a system, including at the data level, the fusion level, and the decision level. Basic functions in sensor fusion implementations include smoothing and filtering sensor data and predicting sensor and system states.
At Renesas Electronics, we invite you to take advantage of our high-performance MCUs and A&P portfolio combined with a complete SW platform providing targeted deep learning models and tools to build next generation sensor fusion solutions.