About Endpoint Intelligence
In the information technology (IT) world, in addition to cloud computing that realizes maximization, investment in edge computing that realizes optimization through decentralization is actively being carried out. Meanwhile, in the world of operational technology (OT), real-time, safety and robustness are worthwhile, not only for improvement of power/performance and productivity but also for the realization of new technologies such as cooperation between people and machines and autonomous control of equipment. The trend is to utilize these two big data concepts by maximizing the cloud and optimizing at the edge. With innovation that begins with endpoint intelligence, Renesas aims to contribute to the realization of an environmentally friendly smart society that supports safer and healthier living.
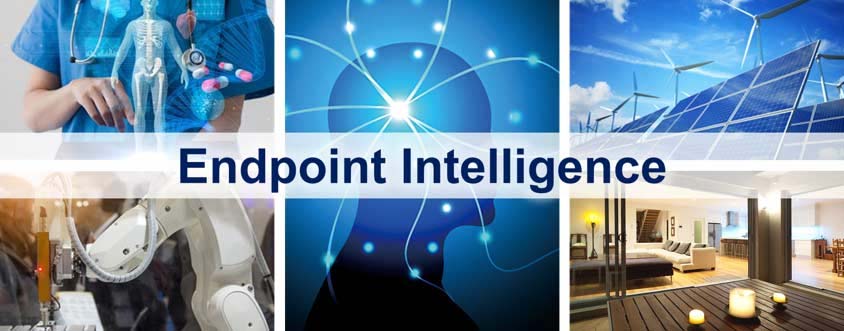
Enhance Endpoint Intelligence by e-AI
The trend to move AI processing from centralized cloud processing platforms to the endpoint is motivated by a variety of reasons including bandwidth constraints, the availability of cloud connectivity, and data privacy. The need for efficient AI inference at the embedded endpoint demands efficient endpoints that can infer, pre-process and filter data in real time. This allows you to optimize device performance and analyze the respective application-specific data points directly at the endpoint while avoiding all the aforementioned constraints. It is particularly effective for abnormality detection and prediction of human behavior and the state of things, and it has already begun to be applied. This is the core of the Renesas e-AI activity.
It is important to understand that embedded AI processing typically means inference processing. In AI terminology, inference is the process in which captured data is analyzed by a pre-trained AI model. In embedded applications, applying a pre-trained model to a specific task is the typical generic use case. In contrast, creating a model is called training (or learning) and requires a completely different scale of processing performance. Therefore, training is typically done by high-performance processing entities, often provided by cloud services. Depending on model complexity, training a model can take minutes, hours, weeks, or even months. e-AI processing does not normally attempt to tackle these kinds of model-creation tasks. Instead, e-AI will help to improve the performance of a device using pre-trained models. Taking advantage of the data generated by rapidly increasing data received from sensors, e-AI can ensure that the device output operates at the ideal state, whether in an industrial drive, a washing machine, or a footstep detector. This is where Renesas focuses - on endpoint intelligence.
e-AI is available for Renesas MCU and MPU products. Additionally, Renesas has developed a dynamically reconfigurable processor (DRP) module, an AI Accelerator, that can flexibly assign resources to accelerate e-AI tasks.
DRP uses highly parallel processing to meet modern AI algorithm demands. As the DRP design is optimized for low power consumption, it can support multiple use cases of customized algorithms with rapid inference which will fit most embedded endpoint requirements. Renesas DRP enables high performance at low power so that it fits ideally into embedded applications. DRP is dynamically reconfigurable, thus allowing the adaption of use cases and/or algorithms within the same hardware.
Renesas e-AI/DRP roadmap includes four performance classes, each class adding 10 times of neural network performance to the previous class. The unique positioning for endpoint inference in combination with Renesas leading MCU/MPU technology gives our customers an unmatched power/performance ratio for AI processing. In the future, we plan to evolve the e-AI solution with DRP technology and realize incremental learning at the endpoint.
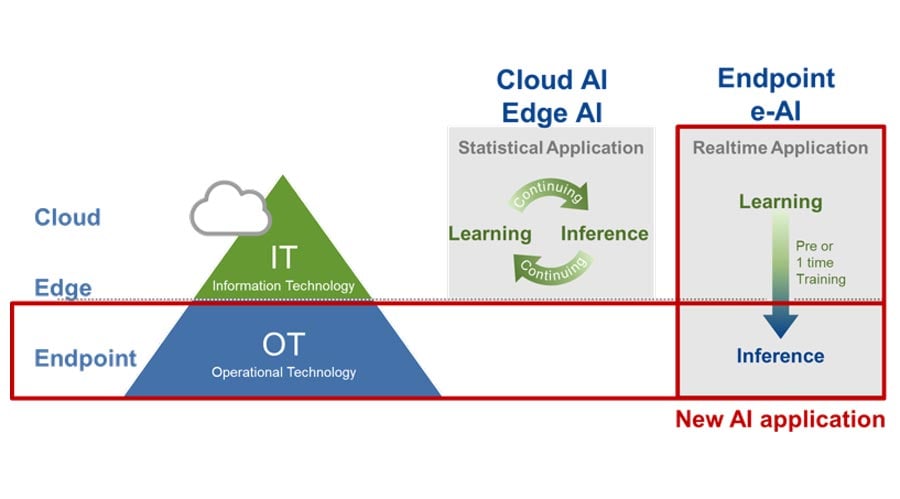
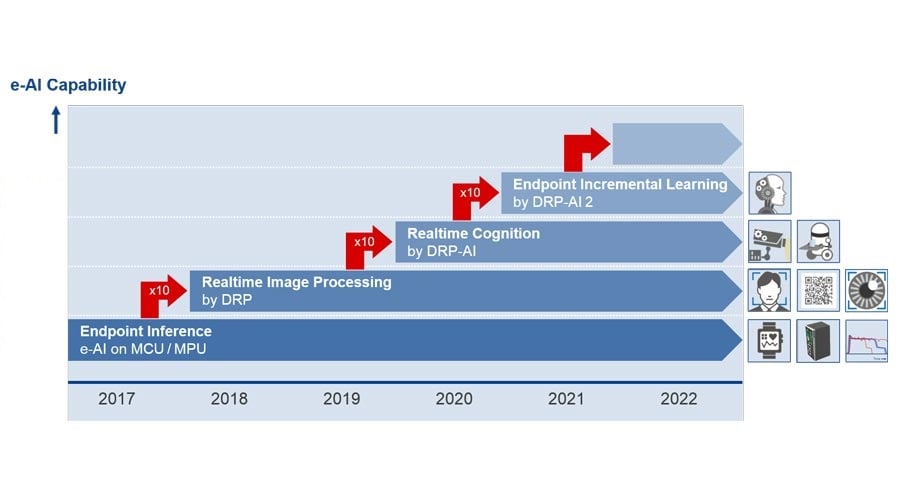